Using artificial intelligence (AI) to track and analyze cyber risks
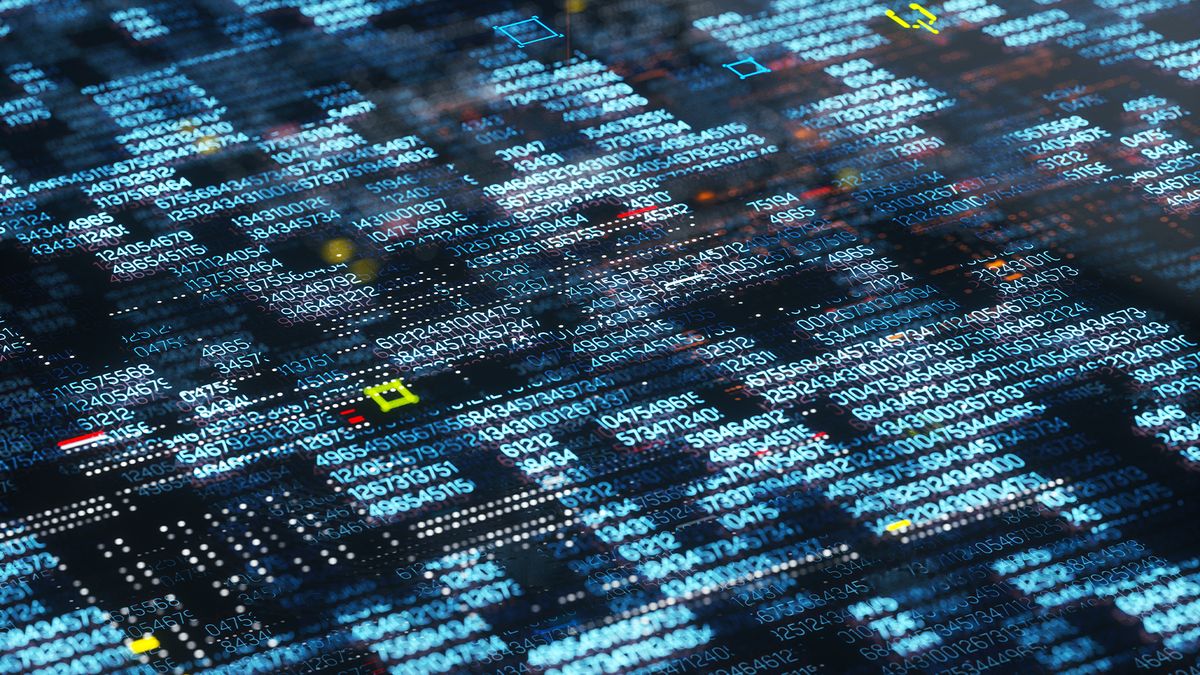
Artificial intelligence (AI) continues to be an instigator of a sea-change for almost every industry it touches, and cyber security is no different. The technology is on the verge of radically transforming the way that organizations and security teams combat the ever-evolving threat landscape.
Although there is a risk of AI becoming something that security professionals are too reliant on – especially while the technology still remains nascent – research is showing the technology is capable of supercharging productivity.
AI is proving especially useful in the tracking and analyzing of cyber risks that an organization faces. And there are a host of new and emerging capabilities out there that can help teams assess the threat landscape specific to their business — from pattern recognition to AI-infused security information and event management (SIEM).
Today’s most prominent risks and threats
Among the top cyber security risks in 2024 are social engineering attacks, third-party exposure, and configuration mistakes, according to research by Embroker.
Social engineering tactics, in particular, were deployed in 74% of all data breaches last year, based on Verizon findings, and social engineering attacks are, unfortunately, becoming more sophisticated. Hackers are becoming more versed in how to trick employees into handing over their credentials, whether through conventional phishing or spoofing, while making their attacks harder to spot.
Cyber criminals are also increasingly targeting the supply chain, with plenty of prominent attacks making headlines this year due to an exposure in a third-party’s attack surface. Simple errors in configuration, too, can be to blame for a great deal of incidents. These may include the failure to change default device configurations, a failure to update or patch systems in a timely way, or simply using weak passwords.
The changing nature of threat detection
The rules-based system of detecting threats that stretches back decades has long been superseded by an AI-based regime in which threat hunting is greatly augmented with the help of machines. It’s been a practical revolution, according to Palo Alto Networks, and a departure from signature-based approaches, heuristic-based threat detection methods, and more recently the anomaly-based detection systems. There was indeed a need for each of these detection approaches, but the rise of machine learning and now generative AI has created a new generation of threat modeling.
Cyber security professionals will inevitably want to adopt AI tools and services for all the strengths these systems offer in areas such as pattern recognition and offering insights – not to mention taking mitigating steps autonomously, according to EC-Council University.
Pattern insights is an area in which AI particularly excels, and has been used broadly across the enterprise, picking out trends that humans may otherwise struggle to spot. When it comes to cyber security, AI may help to spot anomalies that are so buried they’re otherwise anonymous, flagging them for cyber security professionals to further investigate. Certain systems, based on identified patterns, can also offer guidance on which measures to take depending on the nature of the issues flagged. The most advanced AI agents can even take mitigation measures autonomously – with humans only stepping in to review decisions.
How AI can work to flag risks faster than ever before
To meet the challenge of cyber criminals using AI to leverage new types of attack, the cyber security industry is reacting with force – leaning on AI tools to better protect organizations from an emerging wave of threats. AI solutions can examine threats coming from different systems, while also taking into account threat intelligence and the current status of networks as well as data residing with the organization.
Ultimately, these systems can analyze the threats, make predictions and then prioritize what needs to be handled and when, says Joseph Steinberg, cyber security expert and a member of CompTIA’s Cybersecurity Advisory Council. “That can dramatically improve the security of an organization,” Steinberg adds, “because remember, if one attack gets through because somebody prioritized [incorrectly]… that can lead to a catastrophe.”
Developing a threat detection model is far from easy. It often comprises several layers that require expertise in various areas, including the particular threat landscape faced by the organization to how to implement machine learning systems. One simplified process of building an analytical model to flag risks is to first define the problem, then collect and prepare data, before choosing which parts of the data the AI will focus on.
As for what data to pick out, EC-Council University suggests gathering information on the IT asset inventory, using the latest data on global and industry-specific threats. Next, it’s important to pick the right AI model or algorithm for the intended purpose. The model must then be trained on the data so it can learn how to detect threats, before it’s evaluated and tested so the implementation team knows how to improve it. Finally, the AI model must be implemented and continuously updated to improve its capabilities and efficacy.
With the cyber security industry lacking in staff, it may seem a no-brainer to implement AI tools – and to implement them as quickly as possible. Indeed, adopting AI tools and systems will help to analyze risks and detect threats far quicker and more effectively than humans alone would be able to. But it’s also imperative to implement such systems within the confines of well-developed policy and with robust configuration. The last thing an organization needs when seeking to analyze emerging threats is to inadvertently broaden the attack surface in the process.
Source link